Solar arrays can often experience faults that go undetected for long periods of time, causing generation and revenue losses.
In particular, residential-scale solar projects lack sensing and instrumentation for performance monitoring and fault detection.
Researchers at the University of Massachusetts Amherst addressed this issue and developed SunDown, a computer model for residential solar fault detection.
The model sidesteps instrumentation and sensors, and instead leverages correlations between the power produced by adjacent panels to detect deviations from a panel’s otherwise expected behavior.
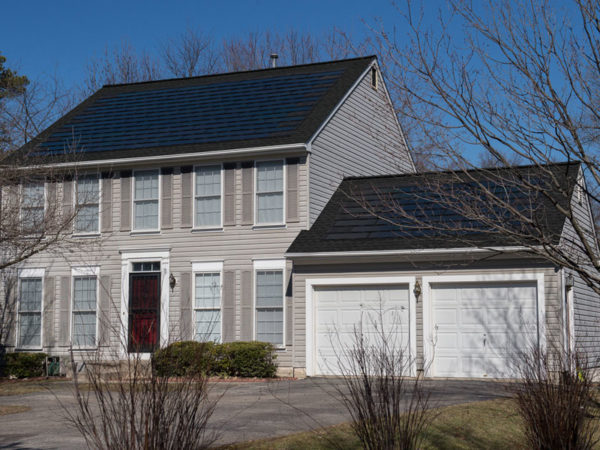
The model can handle concurrent faults in multiple panels and classify the anomaly to determine the probable cause.
SunDown uses two years of solar generation data both from a real home and from a dataset of historic system faults. The result, the researchers said, is a Mean Absolute Percentage Error of 2.98% when predicting per-panel output.
The researchers said the SunDown model has demonstrated an ability to detect and classify faults–including from snow cover, leaves and debris, and electrical failure–with a 99.13% accuracy. It can also detect multiple concurrent faults with a 97.2% accuracy rate.
Two main models are used in SunDown. One is a linear-regression-based model that takes advantage of the high correlation between adjacent panels. The other is a graphical model using what the researchers called “half-sibling regression,” which is based on a recently proposed machine learning technique.
This content is protected by copyright and may not be reused. If you want to cooperate with us and would like to reuse some of our content, please contact: editors@pv-magazine.com.
By submitting this form you agree to pv magazine using your data for the purposes of publishing your comment.
Your personal data will only be disclosed or otherwise transmitted to third parties for the purposes of spam filtering or if this is necessary for technical maintenance of the website. Any other transfer to third parties will not take place unless this is justified on the basis of applicable data protection regulations or if pv magazine is legally obliged to do so.
You may revoke this consent at any time with effect for the future, in which case your personal data will be deleted immediately. Otherwise, your data will be deleted if pv magazine has processed your request or the purpose of data storage is fulfilled.
Further information on data privacy can be found in our Data Protection Policy.