Pairing battery storage with wind turbines or solar panels (either on a site level or utility scale) can add a deeper layer of value to the generation asset. In addition to generation, such hybrid projects facilitate energy shifting applications with rising renewable capacity and changing energy consumption patterns creating opportunities for price arbitrage, allowing developers to shift dispatch to times of higher prices and firming-up capacity commitments sold to the grid.
During the 2021 winter storms, electricity prices in Texas spiked to approximately 300x the median price for 96 consecutive hours, as severe weather and cold temperatures led to restricted access to gas fuel and unavailability of certain conventional and renewable energy sources. For some organizations this resulted in utility bills equivalent to months or years of normal electricity statements in just one month.
Even for companies and organizations with onsite generation assets such as stand-alone solar PV the impacts would have been similar. When combined with on-site generation assets, battery storage systems can be used to ride out the worst of the price volatility – discharging during high prices and absorbing excess energy from generation.
For utility-scale developments, in addition to improving grid flexibility and reliability, co-location can unlock greater value as higher volumes of zero marginal cost renewables are connected to the grid, allowing developers some mitigation of periods of zero or negative prices, depending on the market.
But co-location, either in front or behind the meter, is not the simple proposition it first seems. To successfully unlock the value of both assets requires analysis, interpretation and forecasting of a series of different metrics including utility rates, wholesale energy prices, weather and climate data, site consumption and generation and state of charge and state of health of the battery.
There are a range of grid-balancing services that provide opportunities to earn revenue by supplying stored energy to grid operators. There are also opportunities in some markets to trade energy from battery storage on the wholesale market to capitalize on fluctuating prices. But with multiple markets on which to trade, the investment landscape for battery storage is a complex arena.
Most battery storage owners and operators already know that value stacking is the key to profitability. Batteries are expensive assets, but they can recoup their costs in more ways than one – and using a battery system for multiple applications can greatly improve the asset’s return on investment. Dispatching batteries and then charging them to provide multiple, stacked services creates additional value for all electricity system stakeholders.
With appropriate valuation of those services, such battery business models can also provide net benefit to the system while unlocking multiple revenue streams for the asset owner through the provision of a range of services. But the combined profit potential from participating in ancillary service programs, demand response, or wholesale markets and the non-monetary value in providing backup power also need to be carefully weighed.
GridBeyond’s smart trading solution combines machine learning, AI and data solvers with an extensive trading team; including quantitative and commercial analysts, data scientists and full-time traders. We have long-standing experience managing SOH/ SOC battery assets, and significant investment in data science and accurate forecasting tools, our traders optimize all opportunities with high levels of confidence regardless of size. This will be critical as arbitrage becomes a much larger element of the asset revenue stack.
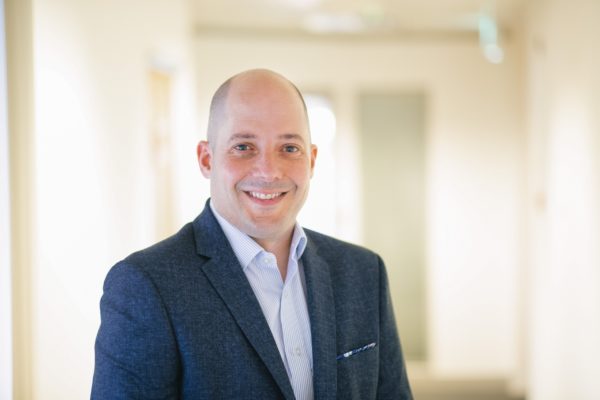
GridBeyond
Chris Smith is the asset development director at GridBeyond. He has been working in the energy industry since 1996. Working with multiple companies at different stages of growth, from start-up to established multi-national businesses. Successfully helping companies to grow their business or market share, predominantly in the purchase of renewable power from generators. His recent focus has been on renewables and ‘Power Purchase Agreements’ and on utility-scale batteries and their optimisation to balance increased intermittency in the power sector.
The views and opinions expressed in this article are the author’s own, and do not necessarily reflect those held by pv magazine.
This content is protected by copyright and may not be reused. If you want to cooperate with us and would like to reuse some of our content, please contact: editors@pv-magazine.com.
I would expect that in order the AI/machine learning to be effective is that it would need access to every consumer endpoint as well as grid junctions. (What % of electrical meters are even smart?) Not only host a database of base demographics, but also historical and real time electrical usage. And, if I dare say, probably the smart thermostat profiles.
Also, since there was planned rotating power outages in 2021 winter storm, then grid profiles to determine which areas were not to be turned off since it hosted emergency or other exception services.
Co -Locating utility scale solar + storage’s battery component close to the actual demand allows two things: It allows transmission of grid scale solar to the battery during off peak times when grid is far less congested.
Second, it allows a reduction in battery size for actual energy delivery to demand since it is discharging directly to the load locally, and the battery itself does not have to account for transmission losses from being located in the desert and discharging during peak grid congestion.
(The issue may be the tax incentives and the co-location rules).